Jan 24, 2024 |
Machine learning-driven prediction of strain engineering in graphene
(Nanowerk News) Two-dimensional (2D) materials like graphene can exhibit exotic quantum mechanical behaviors when subjected to intentional structural distortions. By tweaking interatomic spacings through strain or perturbing electron densities with defects, researchers can finely tune electromechanical characteristics and engineer these atomically thin materials for cutting-edge applications from flexible electronics to quantum computing. However, modeling the intricate quantum structure-property relationships that enable advanced functional design has remained profoundly challenging.
|
Predicting how continuous microscopic strains alter local bond lengths and hopping energies has required computationally taxing physics simulations, frustrating attempts to efficiently scan the astronomical design space.
|
Now, researchers from Zhejiang University in China have developed an ingenious machine learning framework based on generative adversarial networks (GANs) that rapidly predicts atomistic structural relaxations and associated physical fields under continuous strain loading. Their model employs a U-Net architecture for the generative component along with a PatchGAN discriminator. By training on 8000 examples encompassing diverse defect patterns and strain states from molecular simulations, the deep neural network learned to embed strain conditions and generate accurate predictions across held out test cases.
|
While the current framework focuses specifically on graphene systems, the researchers emphasize that the underlying methodology is generalizable to diverse 2D materials like molybdenum disulfide or black phosphorous. Adaptation to three-dimensional bulk crystals also appears feasible, though represents an important direction for future work.
|
Beyond applications in next-generation electronics, strain engineering principles enable emerging routes to reconfigure soft machines and reprogrammable metamaterials via defect actuation. By accelerating fundamental materials predictions, this breakthrough computational approach may soon unlock novel classes of smart mechanical devices previously hindered by modeling and control limitations.
|
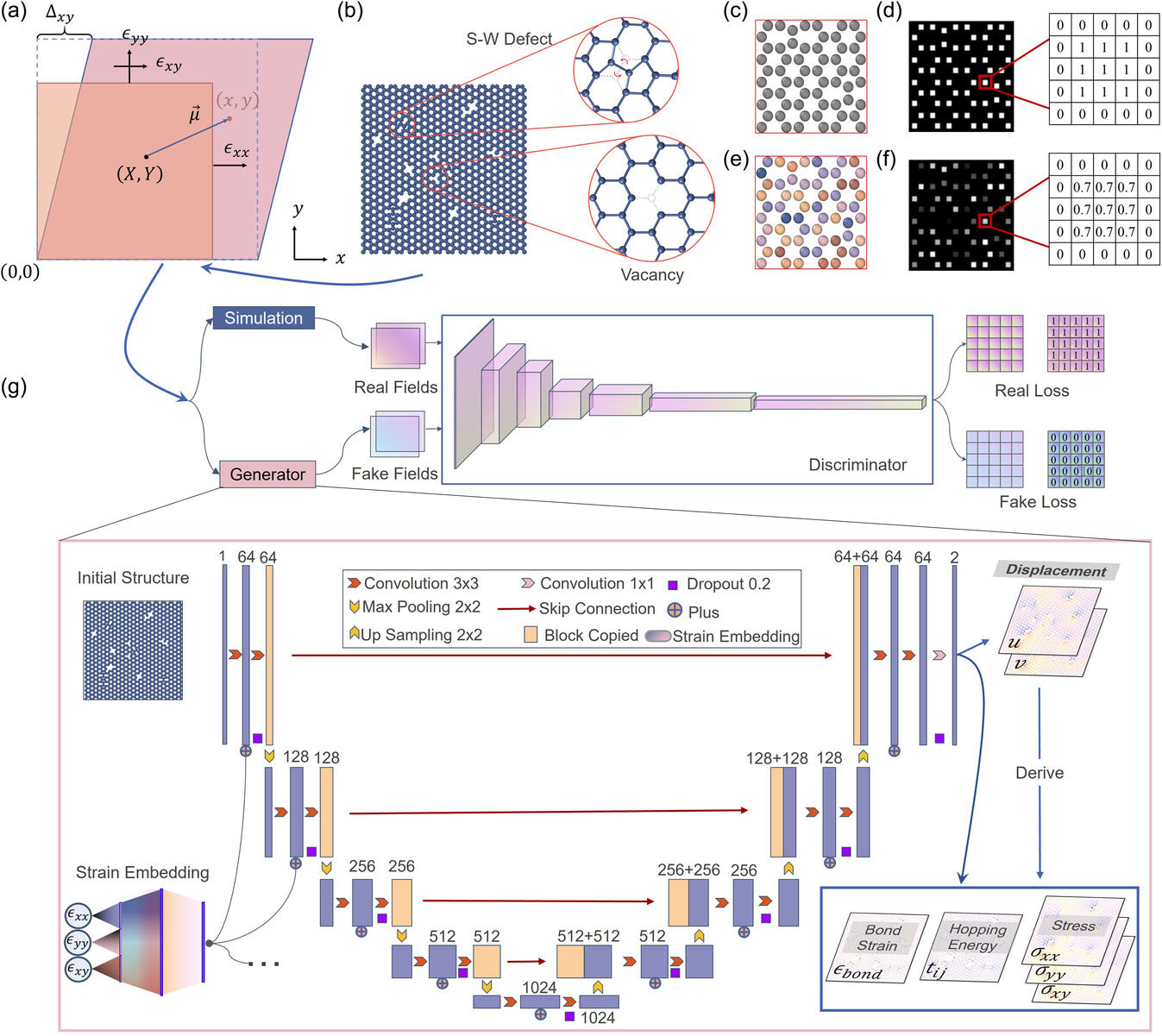 |
Overview of the computational framework in this work. a) The deformation state contains tensile strain (εxx and εyy) and shear strain (εxy, represented by Δxy). A carbon atom with initial coordinates (X, Y) transfers to coordinates (x, y) after deformation with a displacement vector µ. The bottom-left corners of the simulation box before and after deformation are fixed at coordinates (0, 0). b) Illustration on to-be-deformed graphene with Stone–Wales defects and vacancy defects that are studied in this work. c–f ) Brief illustrations on atom-wise pixilation. See main text for elaboration. The atomistic structure (c) is represented by a square matrix tensor (d). The normalized field value (illustrated by different colors) on each atom (e) is presented by a square matrix tensor (f ). g) With initial structure and deformation state, real field images are generated using atomistic simulations, while predicted field images are produced from Generator. The goal of training is to make the predicted image as real as possible as judged by the Discriminator. The deformation state is repeatedly fed into the deep neural network through SE. The work deals with four types of atomistic physical fields that can be directly predicted or derived from the Generator. (© Wiley-VCH Verlag) (click on image to enlarge)
|
The ability to efficiently scan across high-dimensional strain spaces enables researchers to uncover surprising structure-property relationships invisible to conventional techniques. According to author Dr. Shuze Zhu, “this machine learning approach provides an intuitive visualization tool to accelerate discovery of novel quantum materials.”
|
The team reports their findings in Advanced Intelligent Systems ("Deep Learning on Atomistic Physical Fields of Graphene for Strain and Defect Engineering").
|
Their new approach based on generative adversarial networks provides extraordinary accelerations for high-throughput quantum materials screening and offers tantalizing clues toward novel strain engineering pathways unlocked by artificial intelligence.
|
Historically, probing the structure-dependent properties of 2D materials across a multitude of strain and defect states was an extremely computationally expensive task. Traditional simulation techniques like density functional theory (DFT) and molecular dynamics (MD) incur massive computing costs when analyzing the vast number of possible atomistic models. This complexity arises because the design space encompasses countless combinations of defect types, numbers, locations as well as continuously evolving strain loading conditions that alter the equilibrium structures.
|
While machine learning models have shown some promise in accelerating 2D materials discovery, existing techniques have been limited to fixed strain states and lacked the capacity to predict relaxed atomistic structures and spatially resolved physical fields under incremental strain loading. As a result, rapid computational screening and rational design of 2D materials with optimized electronic, thermal and mechanical properties have remained out of reach.
|
To create training data encompassing diverse Stone-Wales (SW) defect patterns and vacancy defects, the researchers performed thousands of molecular relaxation simulations on defect-laden graphene sheets subject to randomized biaxial tensions and shearing using classical potentials. The resulting spatially resolved atomistic displacement fields, bond strains, hopping energies and stress tensor components served as ground truth labels for supervised learning.
|
Remarkably, with only 8000 training examples, the deep neural network could predict various physical fields with R2 scores exceeding 0.97 across held out test cases spanning unexplored strain and defect states. By comparison, traditional simulations required almost 2 minutes per data point. Once trained, the model generated predictions in a mere 0.2 seconds, demonstrating over 30X acceleration. These performance gains pave the way for inexpensive high-throughput screening of strain-engineered 2D materials.
|
But more than just faster simulations, the researchers emphasize that their technique enables qualitative insights difficult to glean from conventional methods. For instance, by analyzing spatial distributions of bond strains and hopping energies, the model reveals surprising deformation hotspots and pseudomagnetic domain formation arising from intricacies of the defect-strain interplay invisible to standard analyses. According to author Dr. Shuze Zhu, “the ability to rapidly scan across continuous strain space under various defect conditions provides researchers with an unprecedented birds-eye view that can unlock new structure-property relationships and accelerate discovery of novel quantum materials.”
|
While the current framework focuses specifically on graphene systems, the underlying methodology is generalizable to diverse 2D materials and nanostructures. Adaptation to 3D bulk crystals also appears feasible but represents an important direction for future work. Beyond applications in next-generation electronics, strain engineering underpins emerging routes to dynamically reconfigure soft machines and reprogrammable metamaterials via defect actuation. This new computational approach may soon unleash novel classes of smart mechanical devices previously hindered by control and modeling limitations.
|
Through ingenious integration of strain embeddings with generative adversarial networks, the researchers have shattered a longstanding barrier that frustrated computational predictions under complex evolving loads central to 2D material design. Their machine learning architecture not only delivers extraordinary accelerations but also provides researchers with an intuitive visualization tool to unlock hidden insights. This breakthrough work underscores the power of physics-informed deep learning and takes a pivotal step toward accelerated discovery of novel quantum materials.
|